252927 Automatic Transmission AL4 DPO Switch Pressure Sensor
Product introduction
1. Common sensor fault diagnosis methods
With the development of science and technology, the methods of sensor fault diagnosis are more and more abundant, which can basically meet the needs of daily use. Specifically, the common sensor fault diagnosis methods mainly include the following:
1.1 Model-based fault diagnosis
The earliest developed model-based sensor fault diagnosis technology takes analytical redundancy instead of physical redundancy as its core idea, and obtains fault information mainly by comparing it with the measured values output by the estimation system. At present, this diagnosis technology can be divided into three categories: parameter estimation-based fault diagnosis method, state-based fault diagnosis method and equivalent space diagnosis method. In general, we define the characteristic parameters of the components that constitute the physical system as matter parameters, and the differential or difference equations that describe the control system as module parameters. When a sensor in the system fails due to damage, failure or performance degradation, it can be directly displayed as the change of material parameters, which in turn causes the change of modulus parameters, which contains all fault information. On the contrary, when the module parameters are known, the change of the parameter can be calculated, so as to determine the size and degree of the sensor fault. At present, model-based sensor diagnosis technology has been widely used, and its research results focus on linear systems, but the research on nonlinear systems needs to be strengthened.
1.2 Knowledge-based fault diagnosis
Different from the above-mentioned fault diagnosis methods, knowledge-based fault diagnosis does not need to establish a mathematical model, which overcomes the shortcomings or defects of model-based fault diagnosis, but lacks a set of mature theoretical support. Among them, artificial neural network method is the representative of knowledge-based fault diagnosis. The so-called artificial neural network is abbreviated as ANN in English, which is based on human understanding of brain neural network and realizes a certain function through artificial construction. Artificial neural network can store information in a distributed way, and realize nonlinear transformation and mapping with the help of network topology and weight distribution. In contrast, artificial neural network method makes up for the deficiency of model-based fault diagnosis in nonlinear systems. However, the artificial neural network method is not perfect, and it only relies on some practical cases, which does not make effective use of the accumulated experience in special fields and is easily influenced by sample selection, so the diagnostic conclusions drawn from it are not interpretable.
Product picture
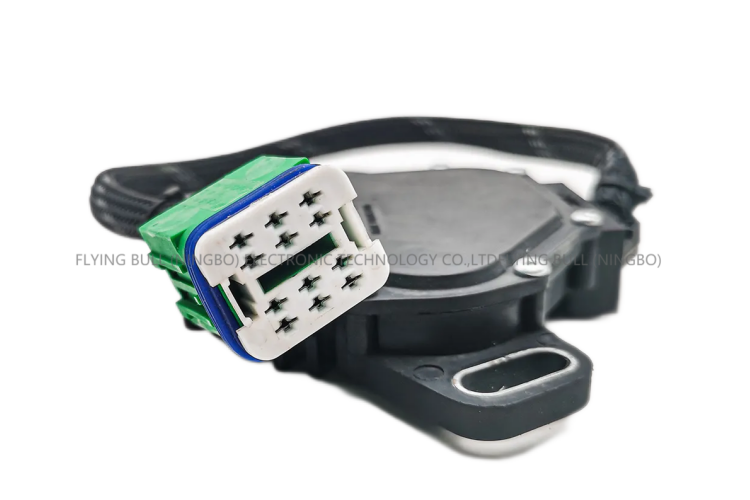
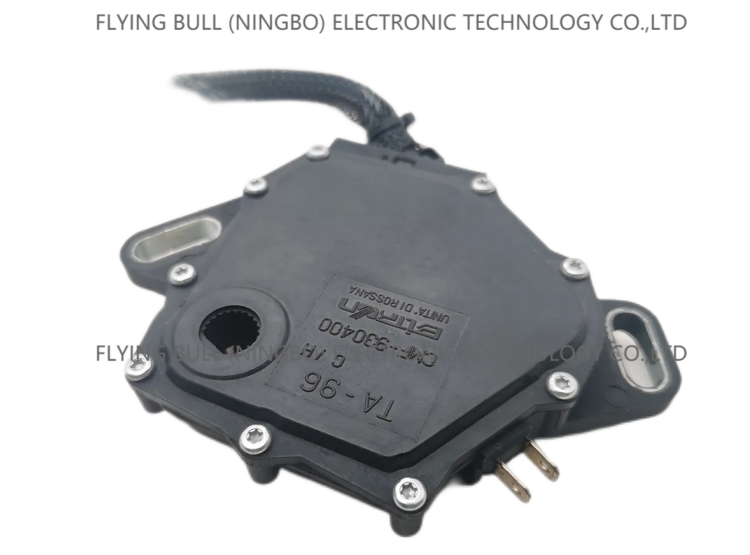
Company details
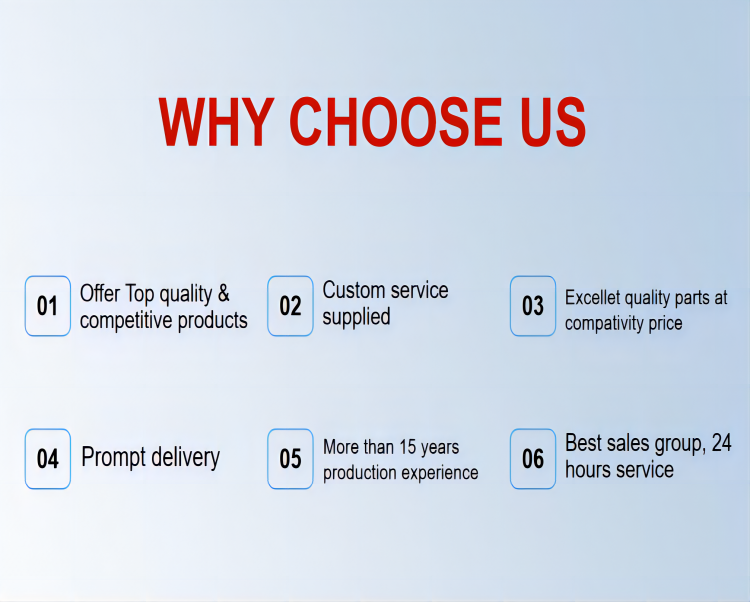
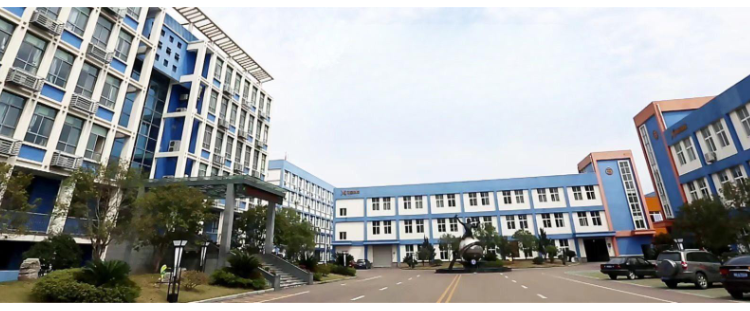
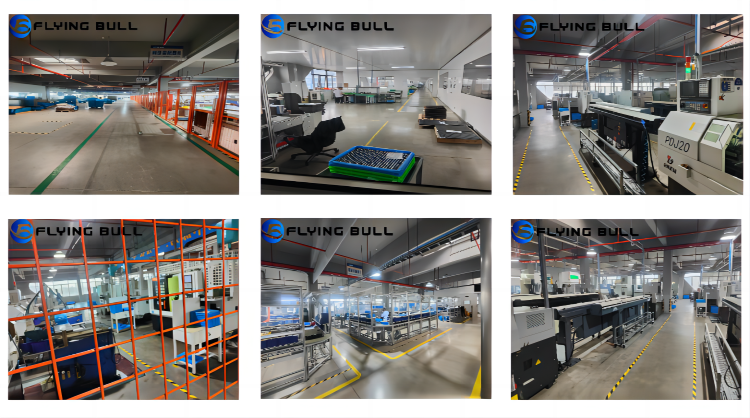
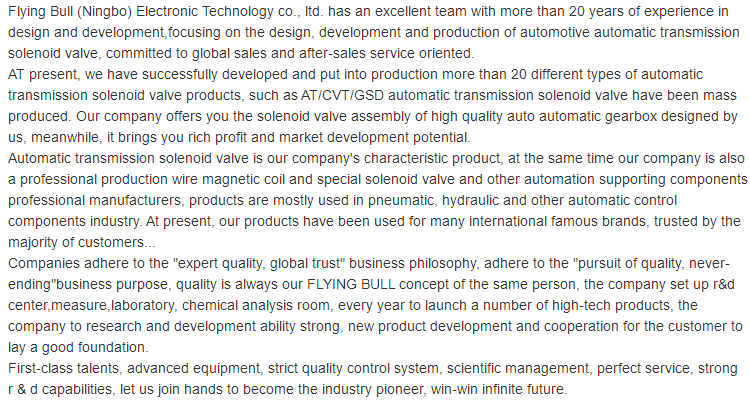
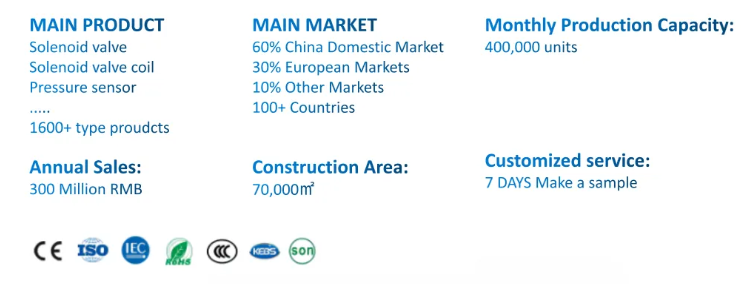
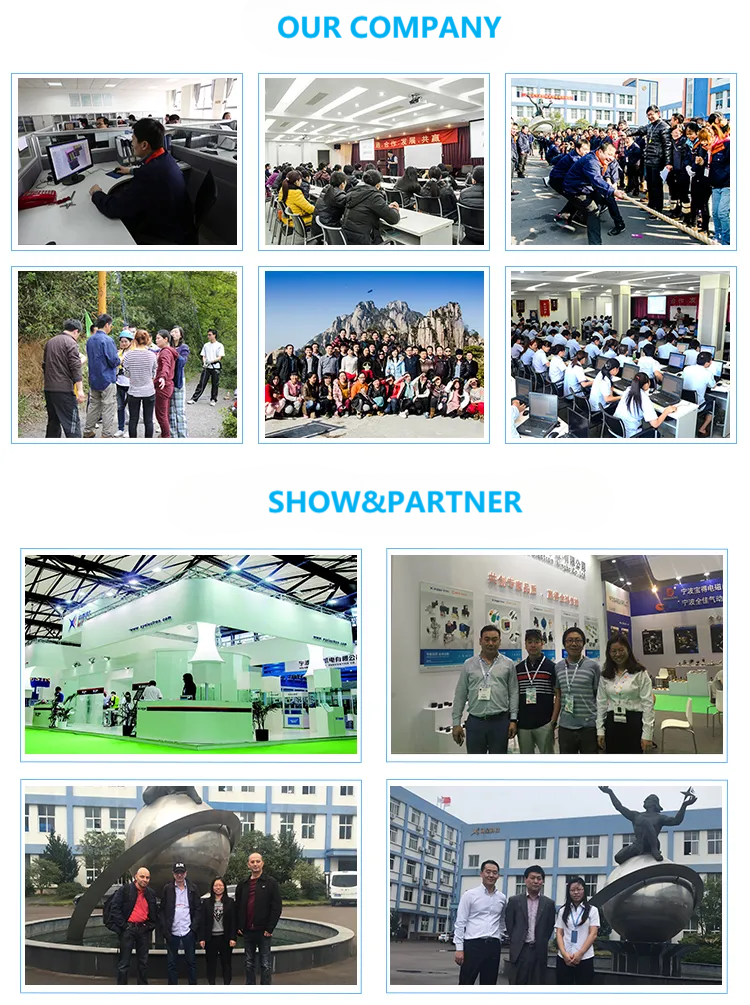
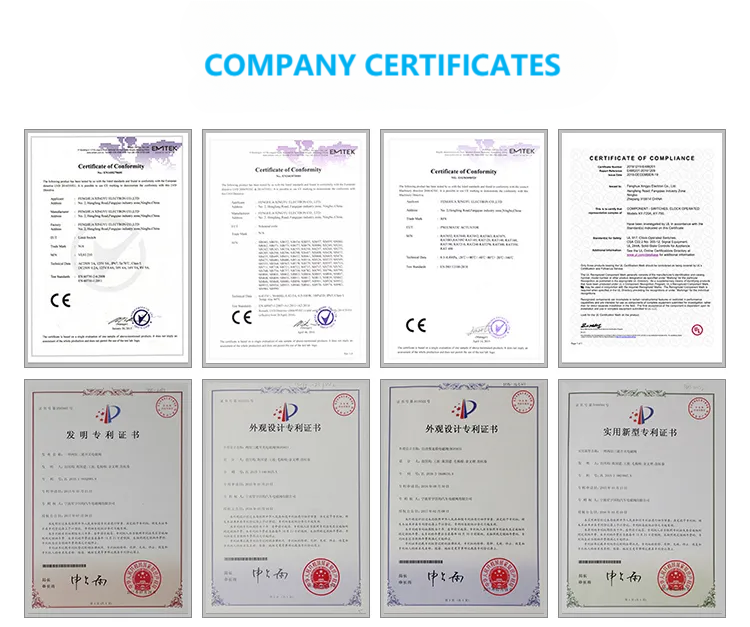
Company advantage
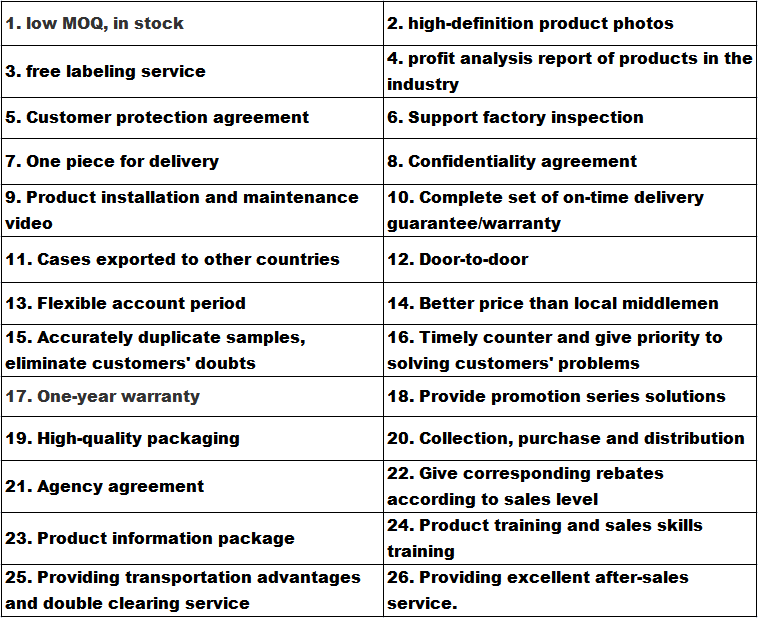
Transportation
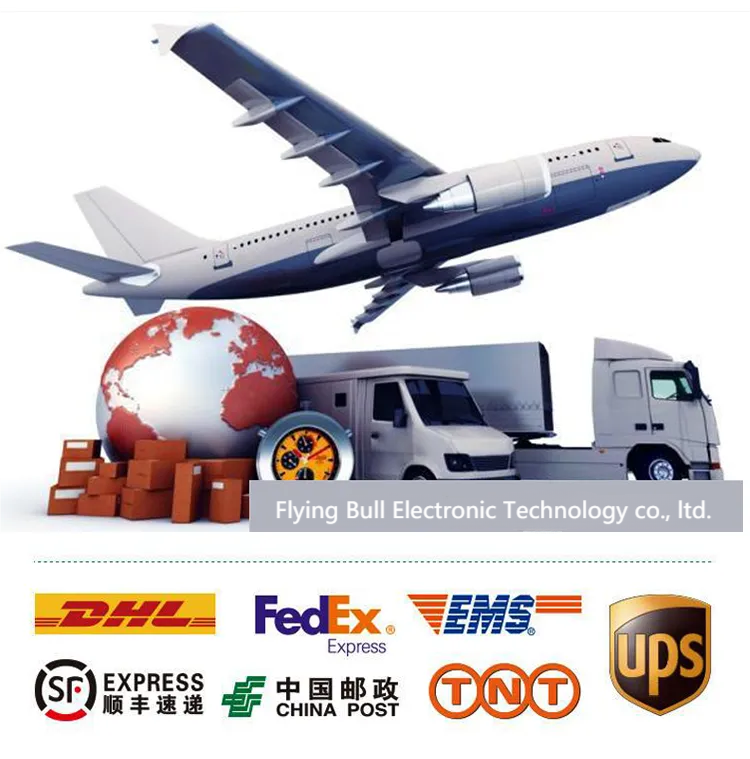
FAQ
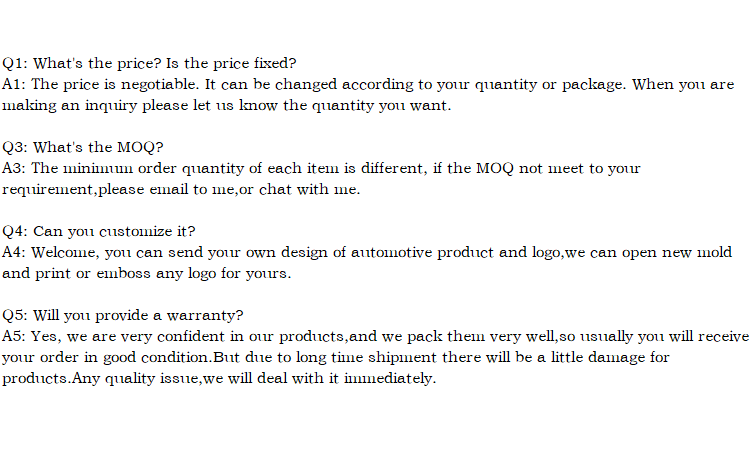